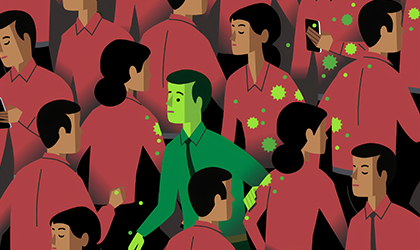
Effective, real-time surveillance is key to the management of the Covid-19 pandemic. In what follows, we first set out a set of principles that may usefully guide real-time surveillance systems for emerging infectious diseases and discuss these in the context of Covid-19. We then briefly review a range of potential sources of data and describe in broad terms strategies for study design and data analysis.
An active surveillance system involves the deliberate collection and analysis of data by sampling from the population at risk according to a specified study design. Passive surveillance involves the analysis of opportunistically collected data. Real-time can mean different things in different contexts; for Covid-19, a reasonable requirement might be that data are collected, and results updated, daily.
A good example of a real-time passive surveillance system for Covid-19 is the analysis of data generated by the Zoe symptom reporting app,1 which covers the whole of the UK. Registered app users are invited to file daily symptom reports, which are then passed through a predictive algorithm and flagged as probable cases or non-cases for further analysis. An ongoing Health Data Research project is using a geostatistical model to generate daily maps of local incidence.2 An animation shows the progress of the Covid-19 epidemic in the English Midlands between 14 and 28 June 2020. Each frame shows the predictive probability, at Lower Super Output Area (LSOA) resolution, that local incidence is greater than national incidence on the day in question, colour coded from dark blue (zero) to bright yellow (one).
Active surveillance avoids the various sources of bias that cannot be ruled out when dealing with opportunistically collected data, but the cost of the associated data collection sets practical limits on the sample size that can be achieved. For example, at the time of writing, the Zoe symptom reporting app has more than four million registered users. Although by no means all users report daily, a continuing active surveillance system with daily reporting of this order of magnitude is unlikely to be affordable. Take, for instance, the national testing survey carried out by the Office for National Statistics (ONS) in partnership with the University of Oxford. A major expansion of this survey is increasing the size from 28,000 people per fortnight to 150,000 by October.
Principles of surveillance
We suggest that any real-time surveillance system needs to take account at least of the following set of principles.
1. Set specific objective(s): One ambitious objective is to predict the pattern of variation in the current incidence of infection for Covid-19 across the UK, either for the whole population or for important subgroups such as particular age or ethnicity groups, using sampled data to understand the current state of a process that cannot be completely observed. A simpler objective is to focus on predicting the pattern of changes in incidence, rather than the absolute level.
2. Use a consistent definition of outcome. This requires either a single diagnostic to be used for case-ascertainment or a way of calibrating across different diagnostic tools. In the case of Covid-19, reverse transcription polymerase chain reaction (RT-PCR) positive tests for SARS-CoV-2 are universally being used to measure current infection. It is known that such tests can fail to diagnose infected people depending on how they are administered and at what stage of the disease process the individual is. This could create additional unstructured heterogeneity but does not necessarily prevent the prediction of changes in incidence.
3. Identify major sources of heterogeneity in risk. In some sub-populations, notably care homes and hospitals, the patterns of exposure and risk are so different from those of the population at large that they are better treated as separate populations, each with their own surveillance system. Similarly, health workers need separate consideration. For the remainder of the population it will be advantageous, both operationally and for statistical efficiency, to stratify by known sources of heterogeneity, for example by population density, deprivation and geographical region. Known individual level sources of heterogeneity, for which adjustment should be made at the analysis stage, include age, sex and ethnicity. Any residual variation in incidence can be considered as a proxy for all unknown, spatially or temporally structured sources of heterogeneity, which will be captured at the analysis phase. We will focus our discussion here on the general population.
4. Sample at random. Only a randomised sampling framework can guarantee unbiased predictions of incidence. A stratified random sample of the UK population, with strata defined by the major sub-population risk-groups may, however, be an unattainable ideal. Post-stratification and adjustment might be sufficient to construct context-specific sampling instruments that can be regarded as “as-if-random.” For example, if an opportunistic sample is post-stratified by age, sex and demography, the within-stratum samples sizes can be compared with national statistics, and within-stratum incidences weighted accordingly.
5. Choose spatial and temporal scales for analysis and for reporting. Incidence for infectious diseases is likely to vary continuously in time and space as a result of both inherent heterogeneity of exposure and the person to person transmission process. In Covid-19, additional spatial clustering might also be expected in relation to super-spreading events linked to a localised context.3 Surveillance data should be recorded and analysed at the finest substantively relevant temporal and spatial resolutions, to facilitate timely decision-making and to avoid spatial aggregation bias. In the current context, the finest relevant temporal scale would be daily, using a rapid-response diagnostic. Some sources of data may be susceptible to weekday/weekend artefacts and adjusted accordingly in the analysis – a persistent feature of publicly reported Covid-19 incidence using unadjusted data has been a spurious drop in the numbers of confirmed cases at weekends. The finest relevant spatial scale in England is likely to be LSOA, used in the 2011 census and numbering 34,753. Several of the known sources of heterogeneity (ethnicity, deprivation) can vary substantially between adjacent LSOAs, and their effects would be masked if data were aggregated to larger spatial units. In Scotland, the equivalent to an LSOA is a Data Zone (DZ).
Currently, in England positive swab tests are reported weekly by Public Health England (PHE) at the level of the 188 Lower Tier Local Authorities (LTLAs). Unfortunately, the number of tests carried out is not easily accessible for the same time and geographical breakdown, hindering estimation of the probability of infection. Reporting positivity rate per 100,000 population can be misleading as it assumes that the testing effort is the same everywhere, which is manifestly not the case.
6. Measure and report degree of uncertainty in predictions. A conventional measure of statistical precision is the standard error of an estimate. In disease surveillance, arguably a more relevant measure is a predictive probability interval expressing the probability, given the observed data, that the underlying process is in a specified state; for example, that the incidence of Covid-19 amongst white females aged 70 or more at a particular location is at least 10%.
Data sources
There are a number of purposely designed surveys, such as the Imperial College REACT study4 and the Oxford-ONS surveillance study, which use RT-PCR swab tests. Additionally, a much larger number of RT-PCR tests are carried out in the wider community (to the exclusion of those with a clinical need and health workers) and reported daily under the heading Pillar 2. The sampling base for the tests carried out in Pillar 2 is not known.
Other routinely collected data sources can provide useful surrogates and contribute to agile surveillance if analysed appropriately. One broad class of routinely recorded data is based on self-reported symptoms. These can be captured by a variety of systems, including the aforementioned Zoe symptom reporting app,1 calls to NHS 111, or indicators monitored by PHE as part of its network of surveillance systems. Symptom-based self-reported indicators typically have lower sensitivity (true positive rate) and specificity (true negative rate) than RT-PCR diagnostic tests and may suffer from biases, which have to be discussed at the analysis phase. Nevertheless, they may contribute agile sources of data which could be integrated as part of a comprehensive system to monitor changes in incidence.
Design
Here, we discuss the “ideal” design of a randomised sampling survey aimed at measuring incidence. For example, the REACT study uses a sample of 100,000 adults over 18 years of age, drawn at random from the population of England.4 We assume that a method of locating a random sample of individuals has been agreed, the choice of diagnostic has been made and its performance characteristics are well understood. The remaining design considerations are: the selection of strata based on combinations of sub-population characteristics; the frequency of sampling; the individual-level characteristics to be recorded on sampled individuals; the sample size(s) to be taken in each stratum. With respect to the last of these, we need either to set a performance target and derive a set of sample sizes that will achieve this, or set an achievable limit on total sample size, optimise their disposition across strata and evaluate the performance of the resulting surveillance system.
Understanding the limitations of a range of affordable designs is more useful than setting a single performance target that is unattainable. Examples of suitable measures of performance could include the maximum width of a 95% predictive interval for LSOA-level incidence within any stratum, or the Receiver Operating Characteristic (ROC) curve for predicting exceedance of a specified incidence threshold at LSOA-level; for an example of the latter, see Fronterre et al.5 As with any sample size calculation, an initial sampling design can only be constructed by assuming a specific statistical model for the underlying spatio-temporal incidence surface. However, data accruing in the early operation of the system can be used to assess the goodness-of-fit of modelling assumptions, to estimate model parameters, and to adapt the sampling design accordingly.
Analysis
Statistical aspects to consider so as to make best use of available data when developing a real-time surveillance system include: (i) how to borrow information from sources of data that may be observational and/or of lower sensitivity and specificity than the target outcome of interest; and (ii) how to take into account spatial and/or temporal correlation in the underlying incidence process.
(i) A relatively small sample of data from a designed study involving a stratified random sample of individuals tested for Covid-19 by RT-PCR at regular time intervals will give unbiased information on incidence level and time trend per stratum, but typically will not have fine enough granularity to deliver reliable estimates of local incidence. On the other hand, if RT-PCR results from swabs of symptomatic individuals who have been tested, say in Pillar 2, are recorded using common strata characteristics as for the designed stratified sampling, then calibration of the routine Pillar 2 testing data with respect to the stratified random sample would become possible. This requires forward planning of the individual characteristics recorded under Pillar 2, as these will condition the design of the stratified random sampling. Under some additional separability assumptions, the geographical granularity of the routine swab test data of Pillar 2 can similarly be anchored by the smaller stratified random sample. This borrowing of information can buy additional precision and adjust for bias in the routinely collected data.
Such a framework could be extended to borrow information from other, routinely collected data of lower sensitivity and specificity with respect to the RT-PCR gold standard. These sources might be more suitable if the goal is to detect local trends in incidence rather than estimate absolute levels. In general, to fuse a variety of data sources in a principled way, it would be important to regularly nest random RT-PCR testing within the lower quality sources, for example the Zoe symptom reporting app discussed earlier or symptom-based reports of NHS 111 calls.
(ii) In a surveillance system of the kind described here, taking account of spatial and/or temporal correlation in the underlying incidence process can materially improve predictive precision, sometimes by an order of magnitude.5 Suitable classes of statistical models for problems of this kind include a generalized linear mixed model6 with either a latent spatially continuous Gaussian process7 or a spatially discrete Markov random field8,9 included in the linear predictor. When several data sources are considered, the borrowing of information described in (i) can be extended to link the underlying spatio-temporal structures. In any statistical model, unexplained variation in the outcome is ascribed to stochastic variation, and the bigger this is, the less precise are the associated model-based predictions. Interventions that are likely to have a major effect on incidence should therefore be included in the underlying statistical model as change-points.
Link between surveillance and the Test-Trace-Isolate (TTI) process
Real-time surveillance and surveillance carried out through active TTI can inform each other in important ways. We believe that quasi-instantaneous exchange of information between the two systems is essential.
The real-time surveillance system estimates the probability that an individual within any area and any stratum is test-positive at a particular point in time. Combining such information with information on the context (e.g. type of work) informs the probability that an individual is at an increased risk of transmitting infection once they have entered the TTI system. This probability gives an objective, quantitative criterion for prioritising the initiation of contact tracing before the test result is returned to the TTI system. Timely questioning to identify contacts in the preceding 14 days could then be done while waiting for the test results. On confirmation of a Covid-19 diagnosis, the TTI system would then be ready actively to trace, and recommend isolation of, all contacts in a timely manner. Cutting the delay between confirmation of an index case and isolation of the contacts is recognised as a key ingredient to make TTI an effective tool for controlling the disease spread.10
Information from the TTI system could then be passed back to the surveillance system so that detecting of potential clusters of cases who are all linked to a specific time and place can be constantly updated and processed using statistical methods that have been developed for discovery of space-time clusters, or “hot-spots”. This topic has an extensive literature dating back to 1964 (and was more recently reviewed by Wakefield, Kelsall and Morris).11,12 Back-tracing from identified hot-spots would be handed back to local public health surveillance teams, to help in the discovery of high-risk contexts or events, for which further specific surveillance activities could be initiated.
Discussion
Real-time surveillance through surveys and TTI are two complementary components of public health action in the face of an emerging threat from an infectious disease. We have outlined how a designed surveillance study can be combined with other routinely collected data sources to create an agile surveillance system that can track the spatial evolution of the disease in real time. We have discussed how steps in the TTI process can be informed by a real-time surveillance system so as to target the contact tracing more effectively. The TTI process can also feedback into the design of specific localised surveillance studies around hot spots.
The general approach is not specific to Covid-19. The current epidemic should act as a spur to the development of real-time public health surveillance systems that can interrogate routinely recorded health outcome data to provide early warnings of anomalies in local incidence patterns for any outcome of potential public health significance. With the recent creation of the Joint Biosecurity Centre, we are confident that the need to design and make operational such a surveillance system is getting increasing traction both in government and in the scientific community at large.
About the authors
Peter J. Diggle is Distinguished University Professor in CHICAS, a teaching and research group within the Lancaster Medical School at Lancaster University working at the interface of statistics, epidemiology and health informatics. He was 2014-16 president of the Royal Statistical Society (RSS), and is a member of the RSS Covid-19 Task Force.
Sylvia Richardson is the Director of the MRC Biostatistics Unit and holds a Research Professorship in the University of Cambridge. She is president-elect of the RSS, and co-chair of the RSS Covid-19 Task Force.
References
- Menni, C., Valdes, A.M., Freidin, M.B., Sudre, C.H., Nguyen, L.H., Drew, D.A., Ganesh, S., Varsavsky, T., Cardoso, M.J., El-Sayed Moustafa, J.S., Visconti, A., Hysi, P., Bowyer, R.C.E., Mangino, M., Falchi, M., Wolf, J., Ourselin, S., Chan, A.T., Steves, C.J., Spector, T.D. (2020) Real-time tracking of self-reported symptoms to predict potential COVID-19. Nature Medicine. doi:10.1038/s41591-020-0916-2 ^
- Fry, R.J., Hollinghurst, J., Stagg, H.R., Thompson, D.A., Fronterre, C., Orton, C., Lyons, R.A. , Ford, D.V., Sheikh, A. and Diggle, P.J. (2020) Real-time spatial health surveillance: mapping the UK COVID-19 epidemic. MedrXiv: https://medrxiv.org/cgi/content/short/2020.08.17.20175117v1 ^
- Frieden, T.R. and Lee, C.T. (2020) Identifying and interrupting superspreading events—implications for control of severe acute respiratory syndrome coronavirus 2. stacks.cdc.gov. ^
- Ward, H., Atchison, C.J., Whitaker, M., Ainslie, K.E.C., Elliott, J., Okell, L.C., Redd, R., Ashby, D., Donnelly, C.A., Barclay, W., Darzi, A., Cooke, G., Riley, S. and Elliott, P. (2020) Antibody prevalence for SARS-CoV-2 in England following first peak of the pandemic: REACT2 study in 100,000 adults. MedrXiv: https://doi.org/10.1101/2020.08.12.20173690 ^
- Fronterre, C., Amoah, B., Giorgi, E., Stanton, M.C. and Diggle, P.J. (2020) Design and analysis of elimination surveys for neglected tropical diseases. Journal of Infectious Diseases, doi:10.1093/infdis/jiz554 ^
- Breslow, N.E. and Clayton, D.G. (1993) Approximate inference in generalized linear mixed models. Journal of the American Statistical Association, 88, 9–25. ^
- Diggle, P.J., Rowlingson, B. and Su, T-L. (2005) Point process methodology for on-line spatio-temporal disease surveillance. Environmetrics, 16, 423–34. ^
- Abellan, J.J., Richardson, S. and Best, N. (2008) Use of space–time models to investigate the stability of patterns of disease. Environmental Health Perspectives, 116(8), 1111–9. ^
- Blangiardo, M., Boulieri, A., Diggle, P., Piel, F. B., Shaddick, G. and Elliott, P. (2020) Advances in spatiotemporal models for non-communicable disease surveillance. International Journal of Epidemiology, 49(Supplement 1), i26–i37. ^
- Ferretti, L., Wymant, C., Kendall, M., Zhao, L., Nurtay, A., Abeler-Dörner, L., Parker, M., Bonsall, D. and Fraser, C. (2020) Quantifying SARS-CoV-2 transmission suggests epidemic control with digital contact tracing. Science, 368(6491). ^
- Knox, G. (1964) The detection of space-time interactions. Applied Statistics, 13, 25-29. ^
- Wakefield, J.C., Kelsall, J.E. and Morris, S.E. (2000) Clustering, cluster detection and spatial variation in risk. In Elliott, P., Wakefield, J.C., Best, N.G. and Briggs, D.J. Spatial Epidemiology, 128-152. Oxford: Oxford University Press. ^