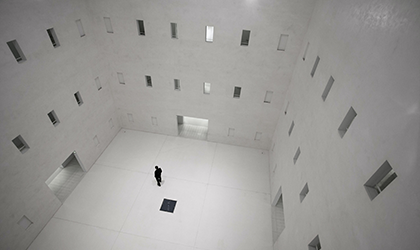
The UK is currently experiencing an infection wave following the co-occurrence of the highly transmissible Delta variant and the relaxation of social distancing measures. Opinions were divided on the wisdom of the decision to relax all social distancing rules in England from 19 July. Without taking a view on the policy, as statisticians we can ask how this decision was made – and in particular, whether a systems view was taken.
This involves acknowledging the dependence between the various metrics we use to measure the health and social impact of the disease, and particularly how those change over time: for example, how the impact of relaxed restrictions will change as disease prevalence increases.
What can we learn from decision theory? It helps us to choose between actions whose consequences are uncertain. In its perfect form, we assign probabilities to events, attribute numerical utility to all consequences of actions and then choose the action with maximum expected utility. Of course, in the real world we cannot confidently do this full technical analysis, but it still gives us a logical thought process in which to initiate a 360º view of consequences of policies.
Let us take the example of the current infection wave. We have seen many models predicting large numbers of cases and, in consequence, an increased burden on the NHS. The government quoted 100,000 cases a day later in the summer. Recent trends have been encouraging, but multiple peaks and troughs in the daily number of positive tests are to be expected in the weeks to come. The transmission environment is highly heterogeneous and influenced by many time-sensitive factors, from evolving immunity to seasonal vacation mobility, from changing working patterns to sporting events. As most epidemic models rely on average contact patterns between groups, they cannot fully account for transmission heterogeneity and the derived predictive scenarios have considerable uncertainties. Faced with this, it is important to communicate clearly how the economic and social consequences of potentially high caseloads have been considered, in particular with respect to the substantial disruption caused by the current contact tracing and isolation policy, which is discussed daily in the press.
Recall that the tracing and isolation policy of the Test and Trace system was set up when the aim was to control the infection rate to a relatively low level by using social distancing as effectively as possible: a very different context to today. The metric “number of people currently isolating” is clearly an important measure of the consequence of a combination of relaxation and transmission control policies. If we are to adapt our transmission control policy effectively to a situation of increased contacts, we need evidence on a range of alternatives. It seems extraordinary that there is still such huge uncertainty about the relative effectiveness of test-to-release compared to 10-day self-isolation orders, when test-to-release policies were discussed in the context of schools as early as December 2020. While a study of schools has just been published, a major trial is still in progress when we desperately need its evidence now.
It is regrettable that much of the discussion does not seem to have taken a 360º view of the systems; instead, we fear that scenarios have narrowly focused on a few metrics. With a focus on cases, hospitalisations and deaths, the impact of knock-on effects from, for example, those having to self-isolate in what has been called “the pingdemic” seemed to have largely been ignored in the presentation of the economic bonus of lifting restrictions. Equally, it would be important that there is a comprehensive discussion on the changing impact of relaxing restrictions if case rates increase substantially in the autumn.
We do not seem to have learnt important lessons from the financial crisis, where the prior financial modelling ignored, for the most part, the dependence between rare events and instead made implausible independence assumptions, with massive consequences that we all experienced. For example, we hope that a current system view does encompass consideration of a metric quantifying the probability that a home-grown vaccine-resistant variant emerges for given levels of population infection and vaccination coverage. To apply rational decision making in the Covid pandemic, we need metrics that cover the whole range of consequences.
Editor’s note
This article has been republished with permission from the Royal Statistical Society (RSS) website.
About the authors
Professor Sylvia Richardson and Professir Sir David Spiegelhalter are chairs of the RSS Covid-19 Task Force.