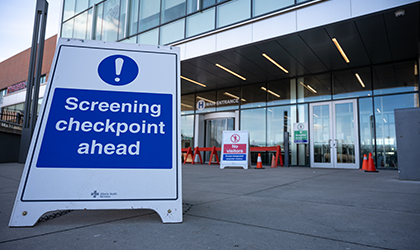
I’m writing this article from the country with more confirmed Covid-19 cases than any other – the US. At the time of finishing my first draft (Monday, 6 April 2020) there were 336,830 confirmed cases. Almost no one, however, believes that this number reflects the true number of Covid-19 cases. Due to the US’s limited testing capacity, we suspect there are cases that have escaped the attention of authorities.
An expansion of testing, it’s been argued, is key to obtaining a better idea of the magnitude of the outbreak. What hasn’t received much attention, however, is the fact that the magnitude of the outbreak affects the quality of testing. To understand this, we need to explain two concepts: the sensitivity and specificity of tests.
The sensitivity of any biomedical test is the probability that a person tests positive given that they have the disease. The specificity of a test is the probability that a person tests negative given that they don’t have the disease.
Using Covid-19 as an example, we can express these concepts through notation:
- P = probability
- Pos = a positive test result
- Neg = a negative test result
- Cov = a person has Covid-19
- NoCov = a person does not have Covid-19
We also use the symbol “|” to mean “given that” or “conditional on”.
With this notation, sensitivity can be expressed as P(Pos |Cov ), which is read as “the probability of a positive test result given that a person has Covid-19”. Specificity can be expressed as P(Neg |NoCov ), which is read as the “the probability of a negative test result given that a person does not have Covid-19”.
Now, the main problems with any diagnostic test are false positives and false negatives. The former occurs when someone without the disease tests positive for it; the latter occurs when someone with the disease tests negative. To see how the quality of a Covid-19 test depends on the magnitude of the outbreak, we need to consider a well-known rule from probability theory called Bayes’ theorem.
This takes the form:
where P(B ) = P(B | A ) x P(A ) + P(B | –A ) x P(-A ), and where –A means “A is not the case”.
In this equation, P(A ) is sometimes called the base rate. The conditional probability P(A | B ) is what we want to find out. Regarding Covid-19, P(A | B ) becomes P(Cov | Pos ). That is, we want to know the probability that a person has Covid-19 given that they have tested positive for it.
Let’s rewrite Bayes’ theorem using the relevant probabilities for Covid-19 testing:
Now, I have a problem: I don’t know the actual figures for the probabilities in this equation as they relate to Covid-19. To start with, the base rate, P(Cov ), is unknown. There is simply no way to know the probability of having Covid-19 – and, conversely, the probability of not having Covid-19 – without a more extensive testing regime. But, for the purposes of the point I want to make, this lack of data isn’t a major problem. In fact, it might actually be considered useful, because my aim is to show how the probability of having Covid-19 given a positive test result depends on numbers about which there is currently some uncertainty (see here and here). So, instead of actual figures, I will use hypothetical numbers.
First, note that the initial expression on the top right side of the equation is the sensitivity of the test. Suppose, for illustrative purposes, we have a test with a sensitivity of 99%. That would mean that 99% of those who have Covid-19 would test positive for it. We write 99% as 0.99 when expressed as a probability.
Next, we have the base rate of Covid-19, P(Cov ) – the estimated prevalence of the disease, which is derived from the number of Covid-19 cases in the US divided by the total US population. At the time of writing (6 April), all we know about the prevalence of Covid-19 is that the US has 336,830 confirmed cases which, when divided by a population of about 329.4 million, gives a P(Cov ) value of 0.1% (0.001). If this were the true population prevalence, it would mean that about 99.9% (0.999) of US residents don’t have the disease, and this gives us our P(NoCov ) value.
One final component: suppose that 1% (0.01) of those who don’t have the disease test positive for it – these constitute our false positives, P(Pos | NoCov ).
We can now fill in Bayes’ theorem:
So, based on these purely hypothetical numbers, and with a purely hypothetical test with sensitivity of 99%, the probability that someone has Covid-19 given that they test positive for it would work out at about 9%. That is, only about 9 of every 100 people who test positive would actually be Covid-19 cases. This implies a lot of false positives.
Now, let’s consider what would happen to our calculation if the number of confirmed Covid-19 cases was underestimated by a factor of 10, as suggested by Dr Dean Blumberg of UC Davis Children’s Hospital, and that instead of 336,830 cases there are really 3,368,300. Our base rate would then increase to about 1% (0.01), and the probably of someone not being infected would decrease to about 99% (0.99). Bayes’ theorem would then yield something like this:
Now our hypothetical probability that someone has Covid-19 given that they test positive for it is about 50%.
And what if we assume that the current estimate of Covid-19 prevalence is off by a factor of 100? This would mean that about 33,683,000 people in the US currently have the disease, bringing prevalence up to about 10% (0.1) and the probability of someone not having the disease down to about 90% (0.9). In this hypothetical scenario, the probability that someone has Covid-19 given that they test positive is about 92%.
By now, we should see the pattern. Even when using a very sensitive test (here 99%), the lower the base rate of the disease the more likely we are to obtain false positives. It can also be shown that the higher the base rate, the more likely we are to obtain false negatives. This is what I meant earlier when I said that the quality of Covid-19 testing depends on the magnitude of the outbreak.
The magnitude of the outbreak is the same as the base rate, and since the base rate appears in the numerator of Bayes’ theorem, P(Cov | Pos ) depends on the magnitude of the outbreak. If the base rate of Covid-19 in the US really is on the low side, we should be prepared for a lot of false positives as we ramp-up testing.
In the midst of a deadly global pandemic, this may be as it should be. Making sure those we believe are negative really are negative may be far more important than making sure those we think are positive really are positive. But we shouldn’t forget that telling people they are positive for a disease when they aren’t comes with a cost. It isn’t just the possibility of being unnecessarily quarantined. It’s also the anxiety of thinking one might have a disease which appears to kill around 1–2% of those who contract it.
About the author
Michael Anthony Lewis is professor in the Silberman School of Social Work at Hunter College, City University of New York. He is also the author of Social Workers Count, published by Oxford University Press.