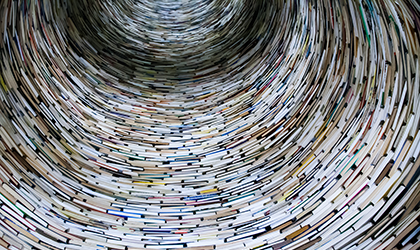
As medical students and budding clinical academics, we have been following developments through the current coronavirus pandemic with interest. We are quite bewildered by the vast amount of data being shared via social media as well as more ‘traditional’ routes such as mainstream media and peer-reviewed journals. This surge in information (of highly variable quality) has been described by the World Health Organization (WHO) as an “infodemic” and has necessitated a “myth busters” section on their website to address the spread of misinformation.
Perhaps one of the most remarkable facts about the current outbreak is the era in which it is occurring. Modern technology has allowed scientific advances to be made at a record pace and the internet age allows rapid dissemination of research findings. The first cases of a pneumonia of unknown cause were reported in Wuhan, China on 31 December 2019. Just one week later, on 7 January 2020, scientists in China had isolated the virus responsible, and its genetic sequence was made available to other scientists around the world just five days later. Now, as we enter the sixth month of the outbreak, a potential vaccine has already begun trials in humans.
Due to the novel nature of Covid-19, the scientific community is conducting research from scratch, albeit drawing experience from other infectious diseases. Faced with this blank canvas, we were interested in examining the scientific response to the early stages of this outbreak. Besides attempting to quantify research output, we can use text mining techniques to identify themes that have emerged from the literature and explore how these themes have changed through the course of the pandemic.
Text mining refers to the concept of drawing meaning from unstructured text and language. Since emerging in the late 1990s, text mining techniques have grown in popularity and use, coinciding with the explosion of data over the past two decades. We first discovered text mining through Julia Silge and David Robinson’s fantastic book on Text Mining in R. We would highly recommend this book for those interested in exploring text mining in a concise and accessible way, and our own analysis is based on several examples from this book, implemented through the tidytext package in R.
In this article, we explore the trends in peer-reviewed publications related to Covid-19 over the first five months of the pandemic, both in terms of the number of articles published and common themes that have emerged.
Data
We chose to focus on peer-reviewed publications published in PubMed up to 31 May 2020. We did not include preprints or articles from other methods of disseminations (such as social media) both for simplicity, and because we were interested in exploring publications that had successfully passed peer review. We identified relevant publications with the search terms “covid”[Title] OR “sars-cov-2”[Title] OR “2019-ncov”[Title] OR “novel coronavirus”[Title]. This search captured some articles related to prior novel coronaviruses, so the search was limited to articles published on or after 1 January 2020. Duplicates (defined as entries with identical titles) were identified and removed.
While PubMed is an established source for obtaining biomedical publications, a few limitations of our search and analysis should be considered from the outset. Covid-19 is a truly global disease and, while PubMed does contain many non-English language publications, it is unlikely that our search has exhaustively captured the full extent of peer-reviewed Covid-19 related literature. Furthermore, we dated articles using their Create Date [CRDT], which describes the date that the citation was added to the PubMed database, rather than the date that the article was published in the respective journal. We did this as the Create Date was easily obtainable from PubMed for all articles in a standardised format, whereas journals differ widely in the way the publication date appears. Therefore, it should be noted that, in some cases, the Create Date and the date of publication may differ by several days or more. Nevertheless, this somewhat crude approach still allows for some interesting observations to be made.
Results
Before we delve into the topics discussed by recent publications, we can look first at the number of Covid-19 related publications over the past five months. Of the 575,052 articles entered into PubMed between 1 January and 31 May this year, 15,779 unique publications were related to the current coronavirus pandemic according to our search terms. While this represents only 2.7% of the total, it implies an extraordinary daily output, as illustrated in Figure 1A. This shows that, since the first article on 19 January, the number of Covid-19 publications per day has grown rapidly on PubMed to a peak of 596 publications per day on 20 May 2020. Up to the end of May, the median number of publications per day was 73 (interquartile range: 19-213). An interesting point to note is that there have been no days since 27 January without Covid-19 related publications. Figure 1B shows the cumulative sum of all Covid-19 related publications published in PubMed. The number of publications grew exponentially from mid-January to mid-February, reaching over 200 publications by 17 February (only 48 days after the first reported cases of Covid-19 from China). Since mid-February, the daily rate of publications has slowed down slightly, though it still increased exponentially until the start of May, where it now appears as though it may be plateauing.
FIGURE 1 Number of Covid-19 publications entered in PubMed (A) per day and (B) cumulatively over time from 1 January 2020 to 31 May 2020. The solid black line in each graph shows a LOESS model fitted to the data. Note that the y-axis is presented on a log10 scale.
With this sizable body of literature, we can learn a lot about the current pandemic through the lens of the vocabulary that these publications use. In an attempt to capture a snapshot of article topics, we have plotted the 100 commonest words from the titles of Covid-19 related publications as a word cloud in Figure 2. Note that we excluded synonyms of Covid-19 from the list as these words are unsurprisingly the commonest. The size of the word is proportional to its frequency with the largest words (such as “pandemic”, “patients” and “disease”) occurring most often.
FIGURE 2 Word cloud showing the top 100 title words from Covid-19 related publications in PubMed from 1 January 2020 to 31 May 2020. Note that synonyms of Covid-19 are not included.
This word cloud gives us an overview of the whole outbreak thus far. The commonest title word of all related publications is “pandemic”. From this, we get a sense that the outbreak is affecting many countries across the world. Furthermore, “China” features frequently amongst titles, suggesting that this country had a large number of cases of the disease. Another country that is commonly mentioned is Italy and, from this, one might infer that they too were heavily impacted by the outbreak. Besides the geography, we can tell a lot about the disease process itself. The tenth commonest word is “pneumonia”, and other related terms such as “respiratory” and “infection” occur often, highlighting that the SARS-CoV-2 virus has a particular affinity for the lungs and presents clinically as a respiratory disease. Moreover, the tone of the terminology used (e.g. “crisis”, “emergency”, “severe”) tells us about the gravity of the situation we find ourselves in.
With this overview in mind, we can zoom in on each individual month to gain a more granular insight into the research topics that are important at various stages throughout this infectious disease outbreak. The term frequency-inverse document frequency (tf-idf) is a widely-used text mining metric used to identify keywords in documents – that is, words that are of specific importance to a particular document within a larger collection.1 It is calculated as follows:
It thus provides a measure of the frequency that a term is used within an individual document, adjusted for how rarely it is used across all documents – and the larger the tf-idf, the more useful the term is as a keyword. In Figure 3, we apply this metric to the title words of Covid-19 related publications, treating each month’s titles as our ‘documents’ and all titles as our ‘collection’, to identify the top 15 words that are characteristic of each month of the outbreak.
FIGURE 3 Comparison of the 15 title words with the highest term frequency-inverse document frequency (tf-idf) for each month of the outbreak. Note that synonyms of Covid-19 are not included as, although these words occur frequently and not in all months, they are relatively uninformative.
In January, we can infer from title words such as “begun”, “tentative”, “originating” and “just” that there was a lot of uncertainty following the first cases of Covid-19 and, already, comparisons were being drawn between this new virus and the SARS epidemic that started in “2002”. There was a realisation that “arduous” times might lie ahead.
In February, key themes show the expansion of knowledge about this virus, with “case” series reporting on its presentation as a “respiratory” disease and the diagnostic utility of “CT” scans in distinguishing between Covid-19 and other respiratory infections. We can also see an emphasis on this outbreak being a “public” health problem, with an essential need for disease “prevention” and “management” of affected patients. Interestingly, the term “what” appears disproportionately frequently in February and March, highlighting how many questions were generated as the world grappled with an emerging disease.
The word “pandemic” is more important from March to May than January and February, and this coincides with the WHO officially declaring Covid-19 a pandemic on 11 March 2020. There also appears to be an increasing focus on “severe” cases in the literature as management strategies were developed and shared for this group of patients. Furthermore, in March, there were more publications on the Covid-19 situation in “Singapore” as cases in the country surged.
In April, there was increasing interest in “hydroxychloroquine” as a possible treatment for Covid-19 and a focus on how Covid-19 would impact the “healthcare” of “cancer” patients and patients who require “surgery”. Similarly, in May, the care of “stroke” patients received particular attention, with a suggestion that Covid-19 can cause neurological symptoms and complications, including stroke.
As we enter the sixth month of the outbreak, publications are now considering what will occur “after” the Covid-19 “era”. In only five months since the first reported cases, well over 15,000 peer-reviewed publications have been published and this number continues to increase. The response of clinicians and academics around the world to this unprecedented global event is inspiring. With social media and online journals, research findings can be disseminated at lightning speed. Many questions have been answered, yet much remains unknown, particularly about the long-term effects of the disease and necessary public health measures on mental health and wellbeing. As the famous adage says: scientia potentia est (knowledge is power). This is especially true when fighting a global pandemic.
Note
Code for this article can be found at github.com/SamuelRNeal/Significance-COVID19.
Acknowledgements
We would like to thank Professor Mario Cortina Borja for his input and guidance when preparing this article.
About the authors
Samuel Neal is a medical student at the University of Aberdeen, currently studying for an MRes in Child Health at the UCL Great Ormond Street Institute of Child Health. His interests include neonatology, paediatric intensive care medicine and global health.
Cindy Zheng is a medical student at the University of Aberdeen, currently studying for a Master of Public Health. Her interests include neurology, infectious diseases and planetary health.
Reference
- Havrlant, L. and Kreinovich, V. (2017) A simple probabilistic explanation of term frequency-inverse document frequency (tf-idf) heuristic (and variations motivated by this explanation). International Journal of General Systems, 46(1), 27-36. ^